Introduction
The AI landscape has been transformed by the emergence of DeepSeek, a groundbreaking model that has caught global attention. DeepSeek’s rise marks a pivotal moment in the ongoing AI race, from its advanced reasoning capabilities to innovative training methodologies. With its roots firmly planted in open-source collaboration, DeepSeek is a technological achievement demonstrating how collective innovation can challenge proprietary dominance.
DeepSeek's R1 was on par with, or in some cases exceeded, OpenAI’s o1 model. It remarkably achieved this feat on a modest $6 million budget. Despite facing challenges like the Biden administration’s AI chip export bans, which restricted access to NVIDIA’s latest chips, DeepSeek delivered exceptional performance using alternative hardware solutions. This accomplishment highlights its ingenuity and the robustness of its cost-effective design.
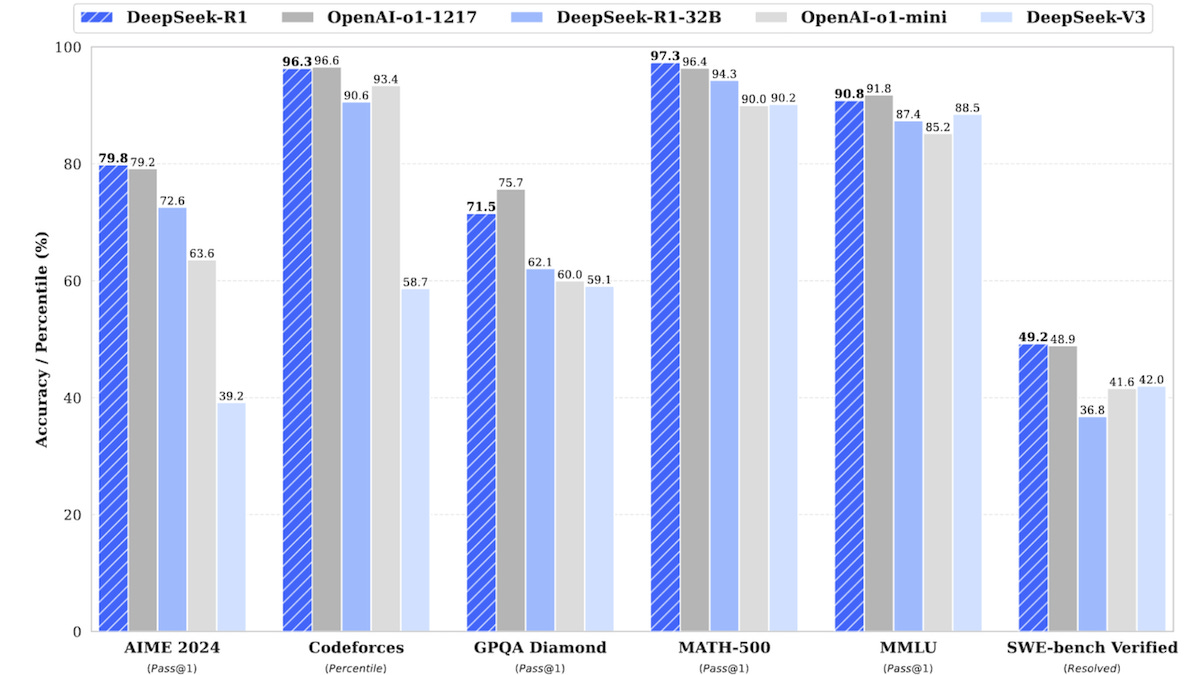
Detailed Advantages of DeepSeek
1. Chain of Thought Reasoning
DeepSeek has excelled in reasoning tasks by utilizing reinforcement learning to improve its chain-of-thought (CoT) capabilities. Through the “Thinking-Out-Loud” approach, DeepSeek articulates the steps taken to solve problems. By explicitly reviewing these steps, the model self-evaluates and can correct mistakes or choose alternative methods to achieve better results. These chain-of-thought strategies have enabled DeepSeek to achieve the same or even higher response accuracy than OpenAI’s.
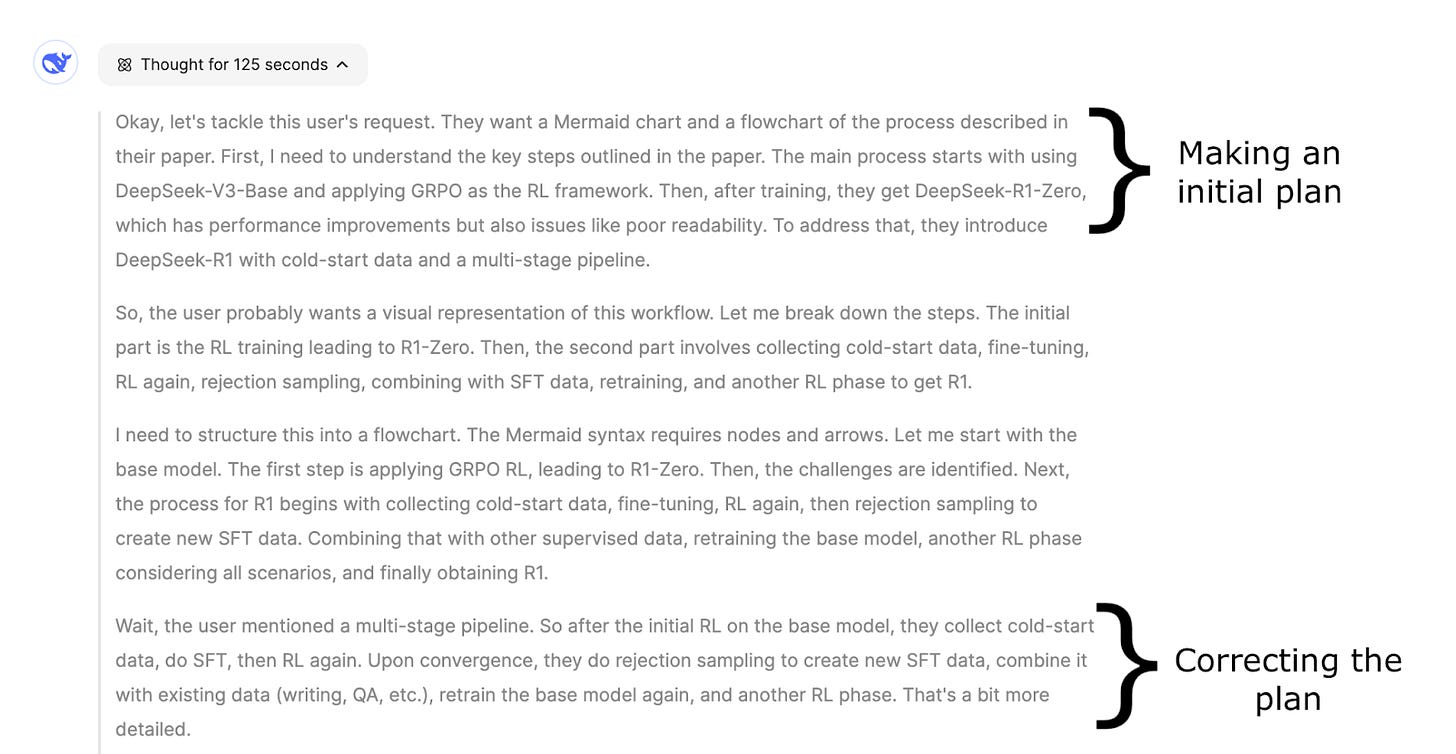
2. Revolutionary Reinforcement Learning Techniques
Unlike traditional models that rely heavily on supervised fine-tuning, DeepSeek introduced a novel reinforcement learning (RL) pipeline in its training. By starting with a "cold start", the model would iteratively refine its ability to solve challenging problems. This is driven by a rule-based system that rewards the model for returning the final answer in a particular format (an accuracy reward) and for showing its internal CoT steps within <think> tags (a format reward). As a result, DeepSeek achieves superior performance while minimizing the need for extensive labeled data that consumes considerable computational resources.
3. Distillation for Broader Accessibility
One of DeepSeek’s most impactful contributions is its ability to distill knowledge from larger models into smaller, more efficient ones. Specifically, a large language model, such as DeepSeek R1 ( 670 Billion Parameters ), serves as the teacher model, providing its advanced reasoning capabilities to guide smaller student models like Llama ( 7 Billion Parameters ). These smaller models mimic the behavior of their larger teachers, maintaining high performance while significantly reducing computational demands. As explained in Good AI’s earlier article on the advantages of small language models, such well-controlled and specialized models form the backbone of cost-effective innovation, democratizing advanced AI capabilities and paving the way for broader applications.
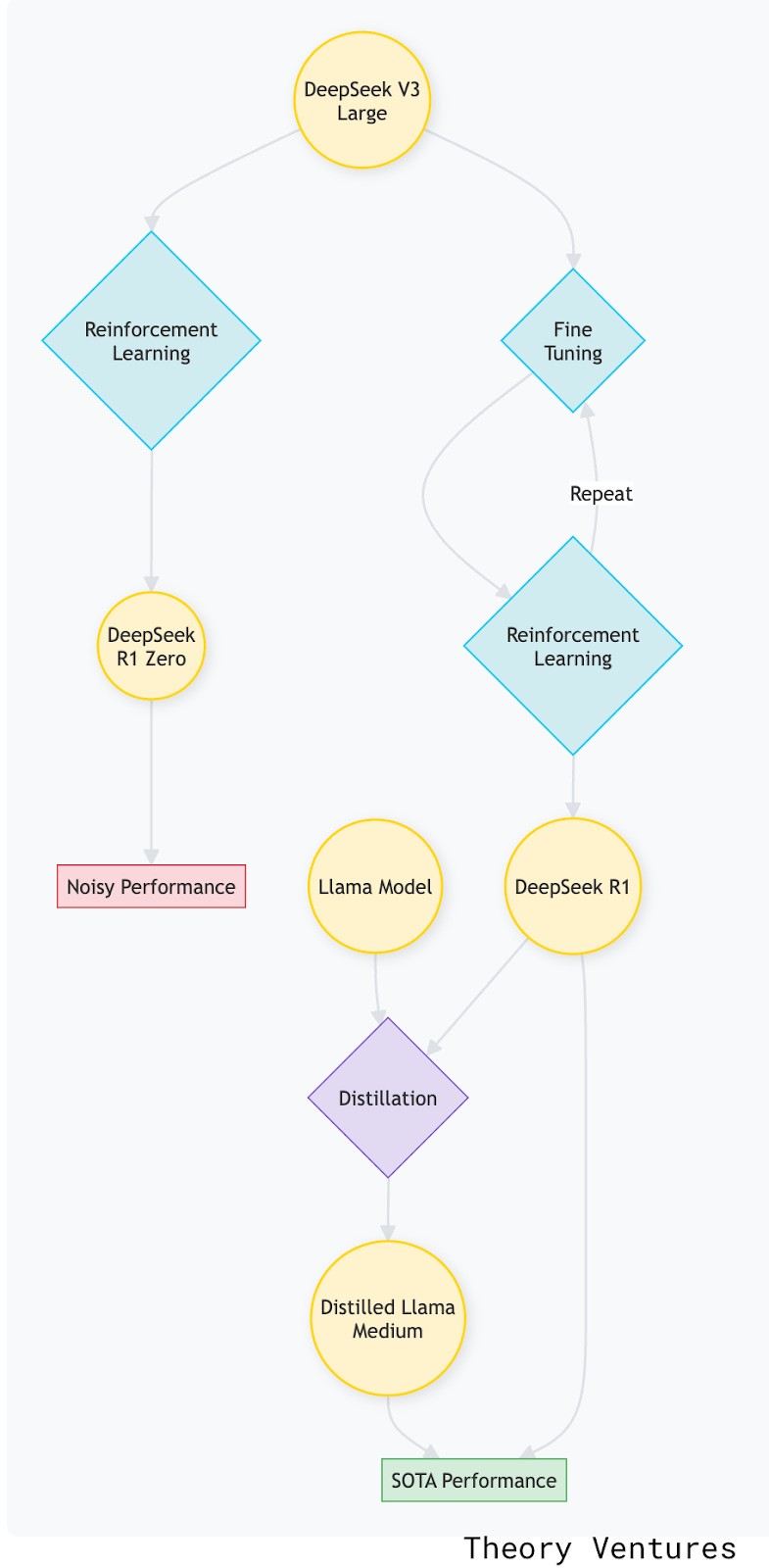
Cost Effectiveness of DeepSeek
DeepSeek’s remarkable performance is achieved with cost efficiencies that set it apart from other AI models. This is made possible through the integration of several advanced methodologies.
1. 8-Bit Floating Point Numbers (FP8)
By replacing traditional 32-bit floating-point numbers (FP32) with 8-bit floating-point numbers (FP8), DeepSeek dramatically reduces memory and computational overhead. This transition maintains performance while significantly lowering costs, as FP8 enables more efficient utilization of hardware resources.
2. Breaking Computations into Small Tiles
DeepSeek employs a tiling strategy, breaking larger computations into smaller, manageable tiles. This approach optimizes memory access patterns and reduces data movement, leading to faster computation and enhanced cost efficiency.
3. Multi-Token Prediction System
The multi-token prediction system is another key innovation. Instead of predicting one token at a time, DeepSeek predicts multiple tokens in parallel, which densifies training signals and accelerates training and inference. This system not only boosts efficiency but also improves overall model performance.
Necessity is the mother of invention
Despite lacking access to NVIDIA's cutting-edge chips, DeepSeek’s ability to accomplish all these within a $6 million budget highlights its resourcefulness. By optimizing older hardware, DeepSeek proves that high-performance AI can be achieved without the latest technology.
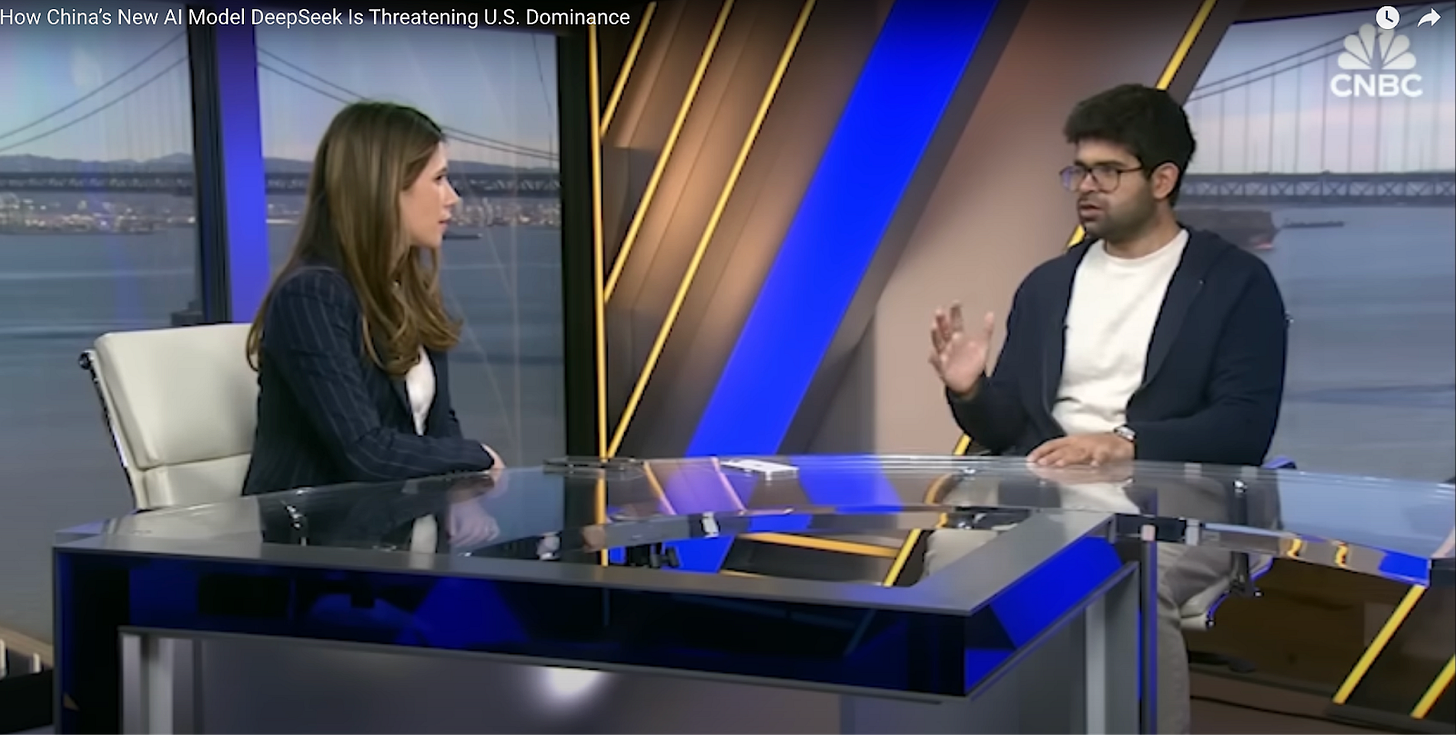
Necessity is the mother of invention; because they had to figure out workarounds, they ended up building something a lot more efficient.
Perplexity CEO Aravind Srinivas on DeepSeek’s resourcefulness
A Victory for Open Source and Inference Compute
1. Open Source vs. Closed Source
DeepSeek’s success is a testament to the power of open-source collaboration. In his LinkedIn post, Meta’s Yann LeCun aptly summarized this shift: “DeepSeek has profited from open research and open source. They came up with new ideas and built them on top of other people’s work. That is the power of open research and open source.”
DeepSeek’s achievements underscore how the open-source ecosystem fosters innovation and levels the playing field against proprietary models from OpenAI and Anthropic.
2. Inference Compute Efficiency
From 8-bit floating point numbers to running a small language model that is as skilled as its larger teacher model, DeepSeek has redefined the efficiency of inference compute.
As Microsoft CEO Satya Nadella noted at the World Economic Forum, DeepSeek’s model is “super-impressive” in optimizing inference-time compute, delivering exceptional performance with reduced computational costs. Such efficiency is pivotal in making AI more sustainable and scalable, particularly in industries like healthcare and finance.
What Comes Next? Innovations and Opportunities
The rise of DeepSeek prompts questions about the future of AI investments. As major players like Stargate, Microsoft, and Meta allocate billions towards data-center-heavy compute infrastructures, DeepSeek’s innovations hint at opportunities beyond traditional paradigms.
1. Decentralized AI Development
NVIDIA’s $3,000 AI developer mini-computer unveiled at CES 2025 represents a shift towards empowering individual developers and smaller organizations. This trend could spark a new wave of innovation outside large data centers, lowering the barriers to entry for AI development.
2. Generative AI Agent Platforms
Salesforce’s AgentForce platform highlights how generative AI can revolutionize customer experiences. Platforms like AgentForce can provide real-time, intelligent solutions that improve business workflows and outcomes by integrating efficient inference models.
3. Healthcare Transformation
During the recent RESI MedTech Panel at JP Morgan, we highlighted the promising trend where AI applications are streamlining practitioner’s workflows. From analyzing full-body MRI scans for cancer to diagnosing liver diseases using ultrasound, AI is poised to recommend actionable insights, improving patient outcomes and experiences. These AI applications, such as stroke patient monitoring systems, must run in an edge environment where inference efficiency is paramount. These innovations are just the beginning of AI’s potential in healthcare.
The application layer is a great place to be
Andrew Ng remarked, “The application layer is a great place to be.” DeepSeek’s impact illustrates the hyper-competitive nature of the foundation model space and the opportunities it creates for application-driven innovation. By combining the strengths of open-source collaboration, inference efficiency, and innovative applications, DeepSeek challenges the status quo and paves the way for a more accessible and sustainable AI future.